
While data analysis helps businesses identify trends and gain insightful knowledge, poor analysis could deprive them of the crucial knowledge they need to grow. However, there is a need to develop a top strategy for sorting and analyzing the data that the company depends on to make informed decisions. Data analytics and automation can be your solution.
So what exactly is data analysis and automation, and why is it better than the traditional way of analyzing data? Read on to find out.
What is Data Analysis?
Data analysis is the process a data expert undergoes to technically gather, clean, organize, and interpret enormous amounts of data. Data analysis is essential for business purposes because it allows the analysis of raw data to make educated decisions based on the services the company provides.
Data analysis helps a business know how well it is doing in satisfying and solving the problems of its customers. And the data used for this purpose come in different structures, formats, and types. Here are some examples of data structures
● The first of these is known as big data, and it has four essential components. These include volume, variety, velocity, and variability.
● Next is structured/unstructured data. Unstructured data is in a format that cannot be organized into rows and columns because it contains different media types, including text, audio, video, and images. Structured data has a predefined data model.
● The third item on the list is metadata, which describes and offers details of other data. The author, image type, and creation date are a few examples of metadata included with an image.
● Real-time data is a different kind of data that is available for use after it is collected. This kind of data is beneficial when making decisions that require current information.
● The next category is machine data, which is automatically produced by machinery, information technology, and telecommunications infrastructure in factories and other settings.
Data analysis methods and techniques
Qualitative and quantitative data analysis techniques are the two main approaches for analyzing data.
● The process of quantitative data analysis entails working with numerical variables, such as statistics, percentages, calculations, measurements, and other data.
● Working with distinctive identifiers like labels and properties and categorical variables like statistics, percentages, and measurements is referred to as using qualitative data.
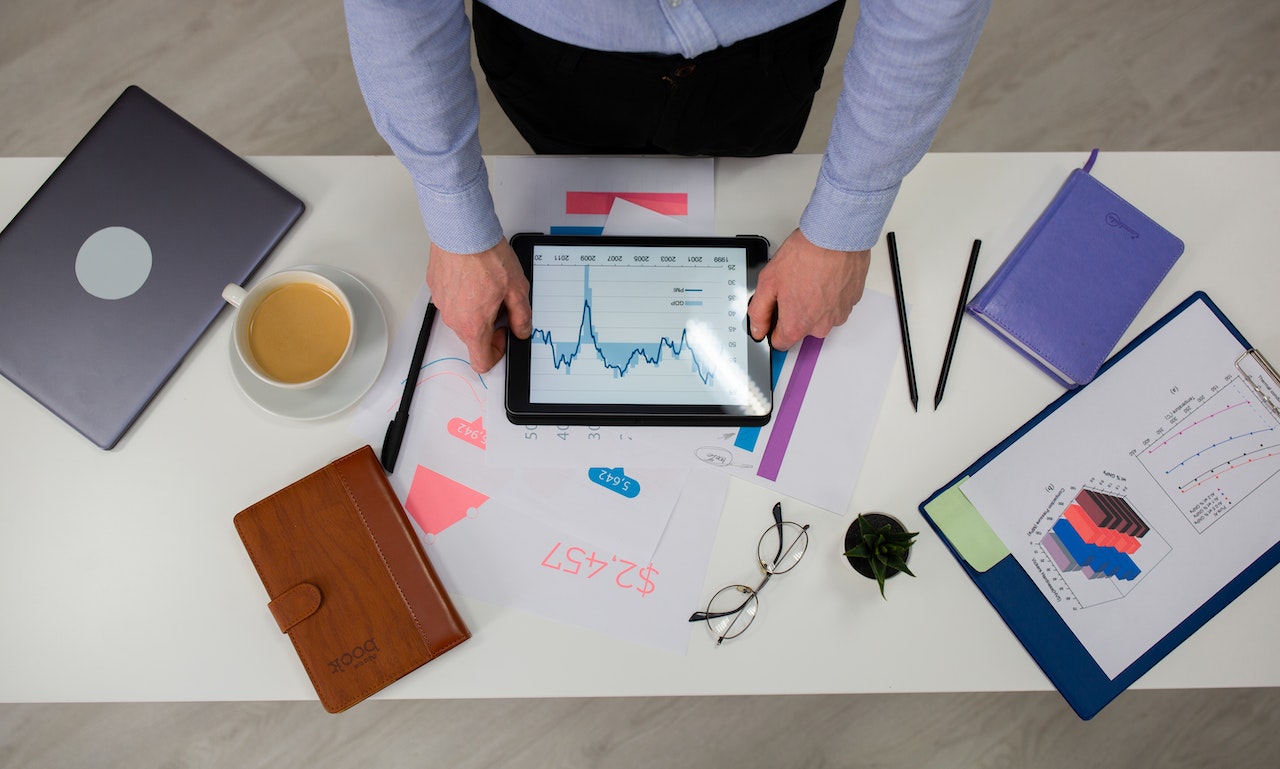
Furthermore, you can independently or combine both to help business leaders and decision-makers acquire business insights from different data types. The traditional way of analyzing raw data is time-consuming and demands spectacular experience and expertise from analysts. The need to quicken the data analysis process and eliminate the manually repetitive and tedious processes demands the need for data analytics automation.
What is Data Analytics Automation?
Automation of data analytics occurs when computers and other analytical tools are used to analyze data. This replaces the repetitive and laborious process of inspecting, cleaning, transforming, and modeling data. Along with many other benefits, it aids in improving customer satisfaction, streamlining operations, and developing more effective marketing strategies.
The monotonous tasks that impede data scientists, analysts, and engineers can affect their output. Automation makes data analysis more flexible and accurate. Running straightforward scripts can change data tables to fit pre-defined data models, requiring little to no human intervention.
Why is Data Analysis and Automation Necessary?
Data is the bedrock of every business, and if correctly analyzed and implemented, it can make a business stand out. Data analysis and automation are reshaping the business landscape and supercharging performance. As a result, new business innovations and competitive models are emerging.
Furthermore, automating the data analysis process allows multiple departments to collaborate on the same data. Each team can support the campaigns and quickly improve where it is falling short, thanks to full access to the data.
For instance, a business that analyzes its data manually frequently spends time analyzing a chunk of the data. And this can affect the business’s growth, as before they get the needed information on where to improve, the customers might have sought similar services elsewhere.
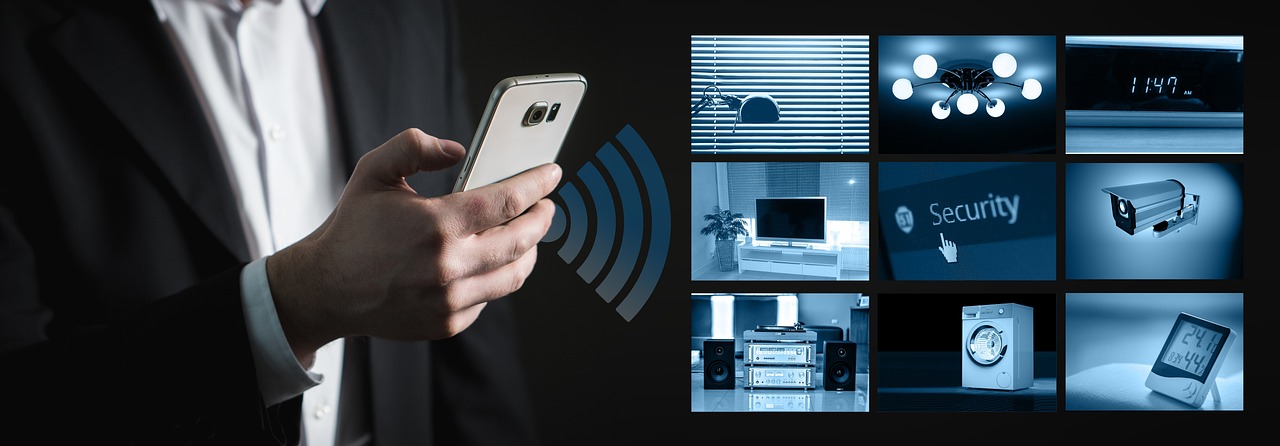
Automation can help analyze data faster and more effectively than the typical exploratory and iterative process. Irrespective of the use case or outcome, automation removes a person from the task and allows a computer to handle it, usually faster and more accurately.
Data analysis and automation can be used for data discovery, preparation, replication, and data warehouse maintenance. They are particularly useful when working with big data. Automation can give a company access to insights that might not otherwise be possible.
Data Analysis and Automation: What are the Benefits?
Without data analytics automation, there would be very high barriers to data access and processing. Automation allows for faster analytics. An analyst can analyze more quickly because computers rapidly perform typically manual or complicated tasks.
Data analytics and automation have far-reaching benefits. Other benefits of data analysis and automation are:
- With automated data analytics, a business can save time and money. Labor costs for data analysis are frequently higher when done manually than they would be if done using technology.
- By automating some tasks where it makes the most sense, data scientists can focus their efforts elsewhere, such as discovering opportunities to find new data sources that can bring new insights.
- It ensures updated, complete, high-quality data without stressing the data scientists.
- Data analytics automation ensures the interpretation of data and the development of new courses of action based on it simultaneously.
- When you automate data analysis, typos, missing values, mismatched formats, and other inconsistencies are avoided. The process ensures data quality and reduces the risk of issues impacting compatibility.
- Errors that cannot be avoided when done manually are reduced through the automation of data analysis. It takes thousands of calculations, hundreds of plots, and hundreds of data points to properly analyze hundreds of test files because humans make mistakes. Data analytics automation completes.
How to Automate Data Analytics
Start by clearly stating and conveying your goals to the board. Then, ensure to involve all relevant parties, including those from operations, sales, marketing, and human resources.
Then, go ahead and define the metrics each stakeholder needs and determine how you plan to obtain those measurements. Afterward, choose your automation tool. Integrating tools that enable machine learning problem detection, automated configuration, and automated predictive and prescriptive analytics is preferable.
After creating a prototype, thoroughly test your automated process to ensure it eliminates rather than adds to manual labor. Then, keep track of the results to see if the data analytics automation is generating expected or unexpected value and if the model can be applied to other domains or use cases.
Remember, there are expert companies helping businesses with data analysis automation. So you can hire these experts to help your business automate the process and save yourself the stress.
Conclusion
Data analysis and automation are best for companies that indulge in routine, rule-based tasks and generate reports. Report generation can take days or even weeks when done manually. Automation can speed up the process while also ensuring that the data is precise, thorough, and even predictive.
Again, automating processes like cleansing a data warehouse can reduce the time spent significantly and guarantee that only the intended data is present. Humans are still required to make decisions, create models, tell the system what to do and when, and properly use the data.